Learning From Voice Conversion Software
How AI voice conversion software helped me better my singing.
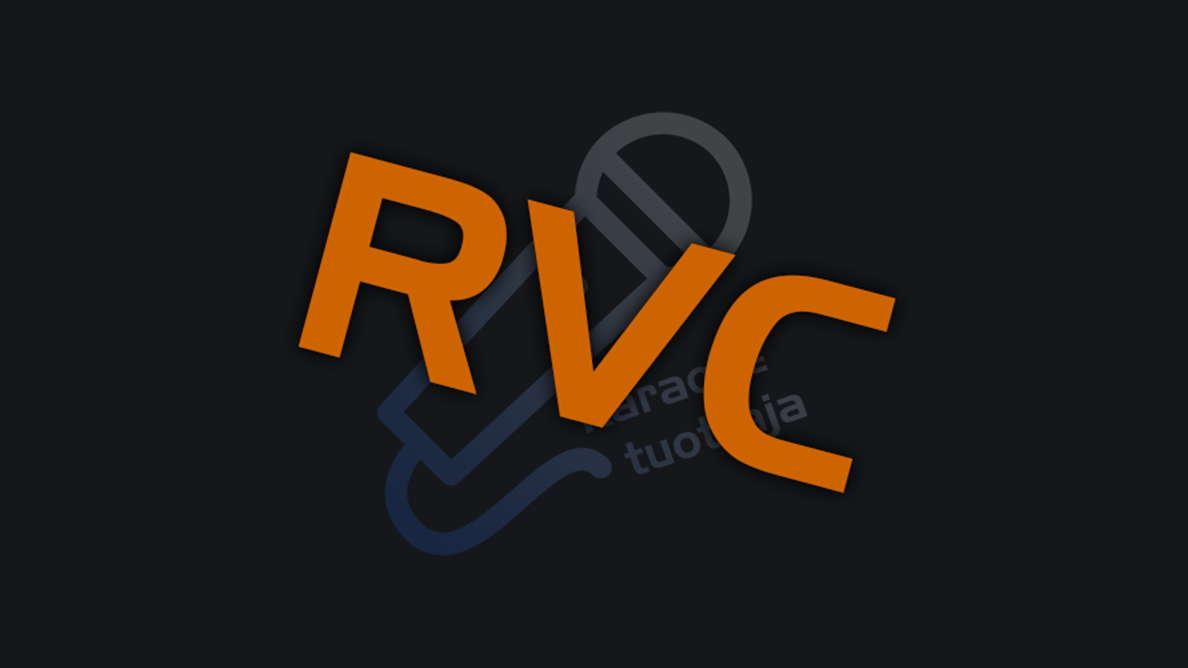
During Christmas 2024 I visited my parents and after some catching up, went to the garage with my dad where he has a karaoke setup with a great sound system. I've been singing karaoke there for I think 4 years now, but this time I wanted to do something different and actually record my voice for later use in some voice conversion experiments.
I wanted to know what my voice sounded like on some songs that I think my voice suits for. These songs are also easy for me to sing, further supporting the theory that I wouldn't have to alter my natural singing voice too much which would hopefully result in good conversion results.
The setup
My karaoke setup deviated from the usual wireless karaoke microphone running through a mixer with sound playback from two speakers to now being my Shure SM58 going through my Audient EVO 4 to my laptop where I was digitally doing the mixing on Ableton Live 11 and then the output sound going from the EVO 4 to my HyperX Cloud II Alpha headphones. By the way, those headphones have been with me for over five years and the audio quality is immaculate; still hearing the differences between lossless and optimally, nearly transparent compressed audio.
I had the microphone input (my voice) with a reverb filter loop back to my headphones along with the karaoke song backing track. Simultaneously and most importantly I was recording the raw microphone input to Audacium to later be used as the dataset for training vocal models of my singing voice.
Recording
Singing in a studio-like environment with headphones compared to the regular karaoke setting with speakers was a bit off-putting at first, but once I started singing, it actually felt pretty nice. You can pick out minute details in your voice when the feedback is right in your ear and you just forget that other people can't hear the music you're listening to at all, which luckily didn't bother me. My dad also made some comments on how it was easier to notice things about my voice he hadn't noticed before with the music blasting from the speakers in addition to my vocals.
Various ranges
I recorded my vocals for 13 songs in Finnish or English from different genres that utilized different ranges of my voice. This would allow me to train vocal models with different tones. I later divided them into these categories:
Low- Jeff Williams ft. Casey Lee Williams - Home (Karaoke)
- Ruth B - Dandelions (lower key) (Karaoke)
- Kari Tapio - Joulun Tarina (Karaoke)
- Ilkka Alanko - Mä En Tiedä Mitään (Karaoke)
- Neljä Ruusua - Pyhäinmiestenpäivä (Karaoke)
- Neljä Ruusua - Tallinnan Aallot (Karaoke)
- Diana Max - Vihree Mies (Karaoke)
- Costi - Maailman Ympäri (Karaoke)
- Neljä Ruusua - Viimeinen Valssi (Karaoke)
- Suurlähettiläät - Kun Tänään Lähden
- Breaking Benjamin - Blow Me Away (Karaoke)
- Pate Mustajärvi - Ajan Päivin, Ajan Öin
- Gloryhammer - Magic Dragon (Karaoke)
Voice conversion models
Retrieval-based Voice Conversion (RVC) is an open source voice conversion AI algorithm that enables realistic speech-to-speech transformations, accurately preserving the intonation and audio characteristics of the original speaker. I knew about this existing, but hadn't looked into the whole process before recording my vocals. Luckily I recorded audio of good quality, which seems to be more important than the quantity of audio.
RVC WebUI installation and setup
For installation and setup of the RVC WebUI, I looked at videos by Nerdy Rodent and TroubleChute which were of great help as the installation process required multiple steps.
Training the models
I prepared the source audio files by cutting out any silent portions in the audio so it would just be useful data per each audio file. I had also normalized the audio right after recording them so the volume was consistent for each clip. The total audio length of each optimized dataset was as follows:
- Low: 00:21:24
- Mid: 00:10:21
- High: 00:08:19
I created three separate models: low, mid and high with the vocal datasets outlined earlier. I trained each model at 100 epochs and the total time to train all of them was about 2 hours with my machine that has an Nvidia RTX 3060 Ti GPU. I was able to get them done efficiently after picking up some useful knowledge from the guide videos and having high quality source files to begin with.
Isolating guide vocals
In order to convert my trained vocal models on another singer's vocals, I needed an isolated track of the vocals. To get this, I got the desired song in lossless quality from lucida using the Deezer or Qobuz service and used UVR Online/x-minus to isolate the vocals. This is also the workflow I use for my Karaoke creation channel on YouTube, Karaoketuottaja.
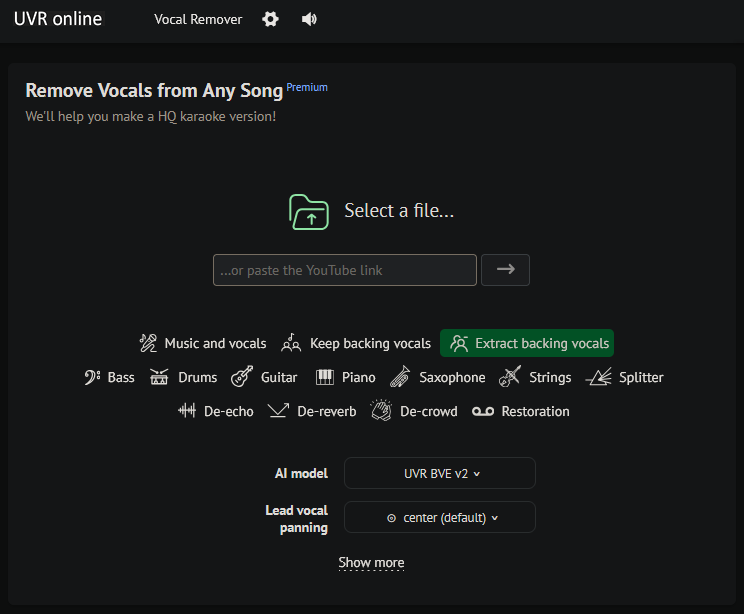
I found that for the best results, you'd want to get the isolated lead vocals with the reverb intact. Having only the lead vocal meant that background vocals wouldn't bleed into the guide vocal and having the reverb present produced more consistent results as artificially removing it would often suddenly dampen parts of sustained vocals in the output.
My process for getting the lead vocals, background vocals and instrumental with x-minus was:
- Lead vocal: Input song file to "Keep backing vocals" with model "Mel-RoFormer (Kar)". Download "Vocals" in flac quality.
- Backing vocals: Input song file to "Extract backing vocals" with model "UVR BVE v2". Download "Backing Vocals" in flac quality.
- Instrumental: Input song file to "Music and vocals" with model "Mel-Roformer OLD (Kim)". Download "Other" in flac quality.
Generating the converted vocals
With the trained voice models and the isolated guide vocals ready, I could combine them to generate a voice similar to mine that follows the guide vocals. As both of these input files were lossless quality, I got the cleanest results possible, which of course was ideal. For each experiment I generated converted lead vocals of the desired song from all my three vocal tone models and compared them in Audacium.
The first experiment I did was with the "low" model using the lead vocals of "Joulun Tarina" by Kari Tapio and was surprised by the results. Having also sung this myself as a part of the source material, I was able to compare their differences:
Comparing the models
Having success with a song I was expecting to be good, I moved on to a different song that I hadn't sung in the dataset; "Rain" by Breaking Benjamin. I was never sure which kind of emphasis on my voice I should use for this song, but luckily I had three models of my voice at different tones to easily compare between and see which one fit the best.
I've compiled samples of this song sung by Benjamin Burnley (original) and the three models of my voice, where each definitely gives it's own vibe. My goal was to see which one fit Ben's voice the best for this song:
The low model sounds more chill and the high model sounds like an epic shout, whereas the mid model is somewhere in the middle like it should be. Using this newly gathered knowledge, I could more accurately decide which model to use on a song cover.
Converted vocal experiments
Armed with the knowledge that my three vocal models performed sufficiently enough, I replaced the vocals of various songs with mine using the methods explained earlier. My goal here was to see if the vocal models of my voice would sound similar to me singing the song for real and also to try and listen for potential mistakes I've been making when singing these songs.
As I have quite a deep voice which I'd say is a minority of the population, it has been difficult trying to research vocal techniques and audio processing targeted specifically towards people with deeper voices. Being able to artificially convert a song's vocals to sound like they're sung by me would allow me to hear what my voice may be capable of in ways that I haven't heard of before. I could also then pick up on the amount of force I need to put into specific sounds to produce them.
I picked three songs where I utilized each vocal model in a way that sounded like they would fit. So using the low model for a chill song and the high model for an epic sounding song. I processed the generated vocals to have similar effects like the original vocals such as compression and reverb, but everything else was left untouched. The following songs are made up of all the isolated tracks just mixed back together into a full ensemble.
Low model song
I picked "Rain" by Breaking Benjamin for the low model as the vocals on this song should not be harsh. The mid model sounded better in some parts, but for the most part I felt like low was appropriate here.
Mid model song
"Memory" by Sugarcult seemed to fit the mid model voice nicely, and I had also enjoyed singing this in karaoke anyways, so I went with it. Some downsides of the model dataset can be heard in this mix as the model can't produce some of the higher sustained notes and also some of the R-sounds are rolled because my dataset for this model was made entirely from Finnish vocals, and in Finnish we always roll the Rs.
High model song
Not going to lie, it was pretty difficult to find a song that suited my voice in the higher model as it's difficult for me to produce those sounds in the first place and a deep voice in a song that's supposed to go hard isn't usually the right combo. In the end I went with "Cry Thunder" by Dragonforce as it seemed to suit my range present in the model while still sounding decent.
What I learned
Doing this deep dive into AI voice conversion showed me examples of a voice that sounds very similar to mine being used in some ways that I hadn't heard of before. Just hearing that allows me to think about how I would produce that sound and as a result better my ability to produce those sounds in a live setting more easily.
I also got a little bit of experience having to process a voice that sounds like mine for it to fit in with the rest of the mix for a song, though I feel it's still far from sounding ideal. I think when recording my vocals in the future, I need to step away from the mic more so the lower frequencies won't be so prominent due to the proximity effect.
I'm also now armed with the knowledge of how to efficiently train and produce vocal models with this Retrieval-based Voice Conversion software allowing me to test it out on some others' voices as well, with their consent of course.